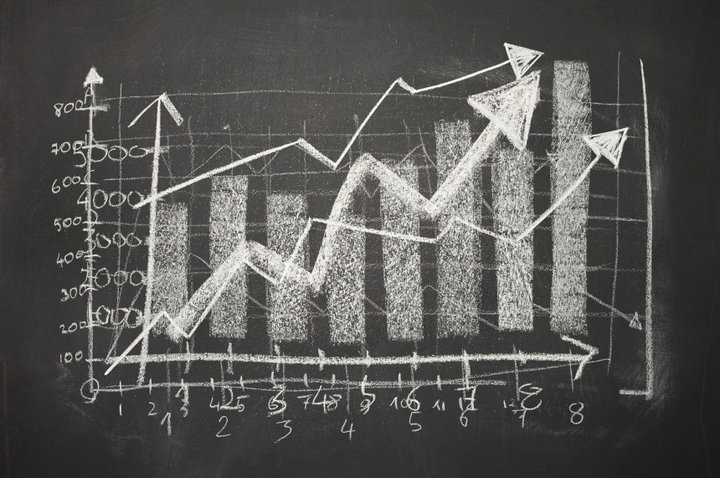
State and federal planners are relying on conflicting coronavirus pandemic models — but an infectious disease model is no oracle. Image via iStock
###
Every day, while we’re all stuck at home, politicians and health officials and news anchors point to graphics showing the latest statistics on the coronavirus pandemic to indicate what might happen next, in your region and around the world.
Underlying those visuals are disease forecasting models — complex mathematical algorithms that predict disease spread and severity based on different scenarios. Because they can help predict the effects of different interventions, including our social distancing, coronavirus pandemic models significantly influence how governments are responding.
It’s tempting to regard these models as oracles that can tell us precisely what to do and when to do it. Can they tell us when we should return to work, or when our kids can go back to school? Is Grandma safe in her nursing home or should she come home? When can businesses safely reopen?
As California’s COVID-19 caseload tops 30,000, state officials are using a model developed at Johns Hopkins University to aid in their planning. If you’ve watched Gov. Gavin Newsom’s daily briefings online, some of the charts shown are based on the model’s predictions.
But the models need to be fed reliable data, and some data is in short supply, especially without widespread U.S. testing for the novel coronavirus that causes COVID-19. We still don’t know how many people have been infected without symptoms, for example. Other inputs, such as incubation periods and death rates, change by the day as we learn more about this virus.
“What makes modeling especially challenging are the human factors. Individual behaviors, health care infrastructure and political response each can affect the outcome of an epidemic,” said Shweta Bansal, an associate professor of biology at Georgetown University who specializes in disease modeling. “I think of models as a call to action. They can tell us what happens if we don’t act and how we can prevent the most dire consequences.”
Here are five things you should know to make sense of infectious disease models:
Why are there so many COVID pandemic models — and why are they all so different?
There are different types of infectious disease models for different purposes. Each has limitations and each can be useful in its own way.
“SEIR” models, for example, involve equations based on the number of susceptible people (S) who can be infected, the number of people exposed (E), the number of people infected (I) and the number of people recovered(R). Agent-based models use massive computer power to simulate the actions of millions of hypothetical people to predict the spread of disease. Still other models examine a disease outbreak in one country and try to predict outcomes elsewhere in the world based on that data. Some models look at travel patterns in spreading disease, and still others assess how age, ethnicity and contributing illnesses may affect survival rates.
Epidemiologists say it helps to have more than one model in responding to disease outbreaks because they use different inputs. Combining results from multiple models can give a more nuanced picture of an outbreak’s trajectory.
“The fact that there are so many models is a healthy sign,” said Bansal. “It’s the same with weather forecasts that rely on multiple mathematical models. But unlike a weather forecast, with a disease forecast we have the ability to change the outcome.”
The White House has controversially used a model developed by the University of Washington’s Institute for Health Metrics and Evaluation, which some disease experts have criticized as being based on overly optimistic assumptions about the benefits of social distancing, among other statistical issues. The Imperial College London model, which predicted as many 22 million U.S. deaths if no action was taken, also apparently prompted the Trump administration to issue tougher new social distancing guidelines.
What goes into making an epidemiological model for an infectious respiratory disease?
California and some other states use an SEIR model developed by Johns Hopkins University epidemiologist Dr. Justin Lessler. This coronavirus pandemic model, according to the California Department of Public Health, takes into account assumptions about how long the disease takes to incubate, how long people are infectious, how many people each patient can infectEp, the fatality rate, how many people need hospitalization or intensive care, and importantly for hospital planning, how many people need ventilators because they can’t breathe on their own.
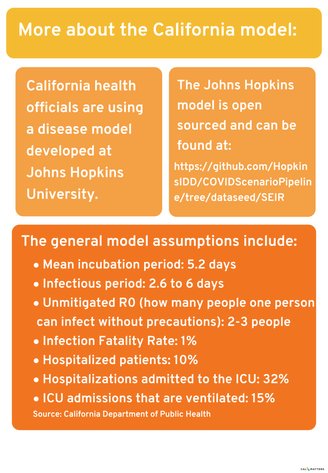
The model assumes that NPIs – “non-pharmaceutical interventions” such as social distancing – started in the state March 20. In fact, some counties ordered residents to shelter in place earlier, some later.
California also has been faring better on a daily basis than this model’s predictions had forecasted — only 4,892 cases were reported on April 16, compared to 12,119 cases projected for that date. Assembly members set aside time at a budget hearing today to question the Newsom administration about the model and seek more details about its assumptions.
Rodger Butler, a spokesman for the California Health and Human Services Agency, cautioned that “there is considerable uncertainty” in the model’s predictions because we still don’t know enough about how the virus behaves and, without widespread testing, how many people are infected. “We are continuously refining our model with researchers and local public health offices,” Butler said.
Which raises the next question:
What happens if you don’t have enough data, or the right data?
This is what keeps public health experts up at night.
Because testing has lagged so badly in the United States, “right now we don’t know how many people are infected,” said Karin Michels, professor and chair of the epidemiology department at UCLA’s School of Public Health. “The biggest unknown (for disease models) is the denominator. How many people out of the infected are actually dying or wind up in the ICU? We have no idea at this point.”
Epidemiologists want to know more about when people are no longer infectious. They hope to learn more about who, once infected, is most likely to need hospitalization or a ventilator, to prevent overwhelming hospitals. True fatality rates aren’t one-size-fits-all; they will differ by age, gender, underlying illness and access to health care, among other factors.
Epidemiologists also need to better understand how many people might have been infected but not show symptoms. New antibody tests now being rolled out can help answer that question, but not quickly, and some of these tests are proving inaccurate. Some public health experts say that preventable delays in widespread testing and a perceived chaotic federal response have prevented the kind of critical data gathering needed to get a handle on outbreaks in various regions of the country — and to provide the kind of intelligence needed before reopening the country.
What makes a model succeed in its predictions?
Disease modelers and mathematicians argue about this a lot. The quality of the data that goes into a disease model is important. The range of error in the model’s predictions are important, too. If early predictions of a model don’t measure up to reality, its later predictions may not as well.
The University of Washington’s coronavirus pandemic model, for example, which aimed to predict outbreaks based primarily on early data from China and later Europe, has had such a wide range of error in its predicted death rates that some epidemiologists have recommended that governments do not use it for planning. The model’s predictions of when cases will peak in various regions also have swung dramatically in different weeks — and rarely coincided with other models’ forecasts, prompting some experts’ concern about the model’s validity going forward. Some politicians are citing the model’s relatively optimistic forecasts as justification to open the country earlier than public health officials recommend.
The model’s developers say they are continually updating their model and have adjusted it to reflect regional differences in how daily death rates peak over time, and how social distancing policies vary by region.
Still, disease models are supposed to serve as a wake-up call prompting us to act. If our actions succeed in slowing infections and deaths, making the predictions inaccurate — that’s a good thing.
What do public health experts wish people understood about coronavirus pandemic models?
Disease models are not perfect, and they can adapt as new data becomes available. Models “can help us think through different scenarios, but they shouldn’t be used as crystal balls,” Bansal said. “If we believe that a model’s prediction of 100,000 deaths is what will happen if we don’t act, then we do everything we can to prevent those 100,000 deaths.”
Also: they’re better for predicting short-term needs and outcomes, Michels said, such as how many ventilators a hospital might need next month, rather than next year:
“I don’t think we can predict the fall yet. We only know the virus will still be around, so life will be complicated.”
###
CALmatters.org is a nonprofit, nonpartisan media venture explaining California policies and politics.